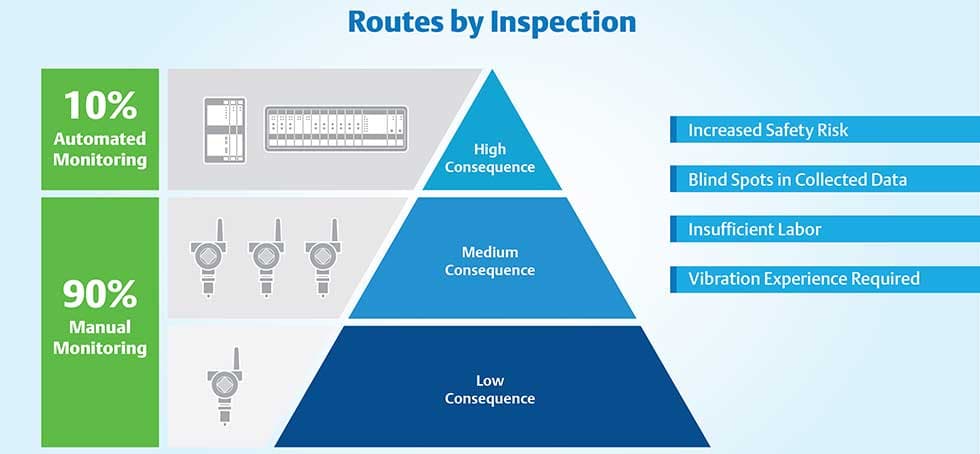
In the mid-1990s, manufacturing plants began to roll out asset monitoring technologies in waves as they recognized the profit hidden in their maintenance departments. Asset monitoring was expensive, so those early rollouts were often limited. Plants defined their assets based on criticality to production, typically using online, rack-mounted systems to provide continuous monitoring for only a select few of the most critical and expensive assets.
For essential assets, these organizations typically relied on “routes by inspection,” a process still common in many plants. Plant personnel collect data from plant medium- and low-consequence assets—either by performing manual rounds with a handheld analyzer or collecting raw data via wireless sensors in places that are not easy to collect with a handheld. They then analyze the data in the main office and ultimately respond to any aberrations.
Today, plants are reconsidering this strategy for several reasons, including high labor content, blind spots between data collection, an inability to cover the entire plant, the need for safety and the decreasing cost of pervasive sensing.
Some assets, such as pumps, are not only essential to the daily operation of industrial processes but are also abundant throughout the plant. These pumps begin to degrade the moment they start up and knowing how to intervene before they fail can potentially save hundreds of thousands of dollars. As part of their digital transformation, high-performing organizations are adopting “routes by exception” for essential assets, automatically collecting data and analyzing health at the device to reduce manual maintenance routes, encourage predictive maintenance and unlock even more profit.
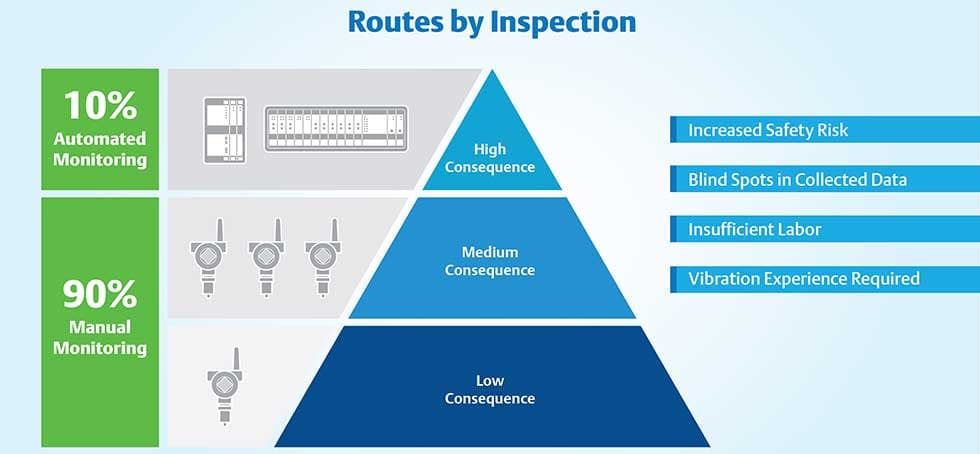
Modernizing Machinery Monitoring
For decades, high-performing plants relied on the criticality pyramid to guide asset monitoring infrastructure decisions. Monitoring technologies were expensive, and the cost and hassle of engineering and wiring equipment made it difficult to justify widespread use of continuous monitoring. Wireless technologies were available, but plants had limited wireless infrastructure, with higher installation costs and limited data transmission capabilities. Plants needed to make decisions about what data was valuable enough to transmit wirelessly.
As a result, automated monitoring was reserved for only the most critical equipment—typically about 10% of the plant’s overall assets. These were the assets posing the highest risk to production, safety and cost and were often the same assets connected to protection systems. However, balance-of-plant equipment was not ignored. Many plants had a bench of personnel who could collect, organize and analyze data.
Today’s plants look different. In the last 10 years, plants have lost experienced personnel to retirement or other facilities. Modern plants are often running smaller, less-experienced teams. The people who used to be spending their days analyzing data collected from balance-of-plant equipment are now analyzing critical asset data. They may even be the ones responsible for performing the inspection rounds necessary to collect that data. As a result, data is being collected less frequently or on fewer assets, leading to blind spots in plant health and a decrease in performance visibility.
Expanding Monitoring
To offset the impact of smaller, less-experienced teams, many organizations are rethinking the criticality pyramid from a digital transformation perspective. At the core of those changes are new digital sensing technologies emerging in the last five years. Increased wireless connectivity and bandwidth, coupled with a wide variety of new, cost-effective sensing options have made it easier for organizations with limited resources and personnel to maintain visibility of a wider range of plant assets. Instead of following the “routes by inspection” model where personnel must travel into the field to collect data, plants are digitally transforming maintenance with new pervasive sensing options to automatically collect data and perform edge or prescriptive analytics and send it directly to the office.
The primary advantage of automated monitoring systems is they enable a safer, more regular and more reliable collection of data. Where traditional route-based monitoring increases risk—like in refineries where collecting vibration data from pumps moving hydrocarbon products often requires personnel to travel to potentially hazardous areas with portable analyzers—automated monitoring reduces the need for such trips. Teams instead install safe, wireless sensing devices so personnel only enter hazardous areas when there is a problem to be solved.
In addition, with automated monitoring in place for all assets, plant personnel spend their time using data rather than collecting it. Time typically spent collecting asset data can instead be spent on the plant floor improving performance and quality. Smaller teams can be more effective, as they do not need to use personnel for data collection and do not have to worry essential information will be missed when a route is skipped due to an absence or all-hands-on-deck activity.
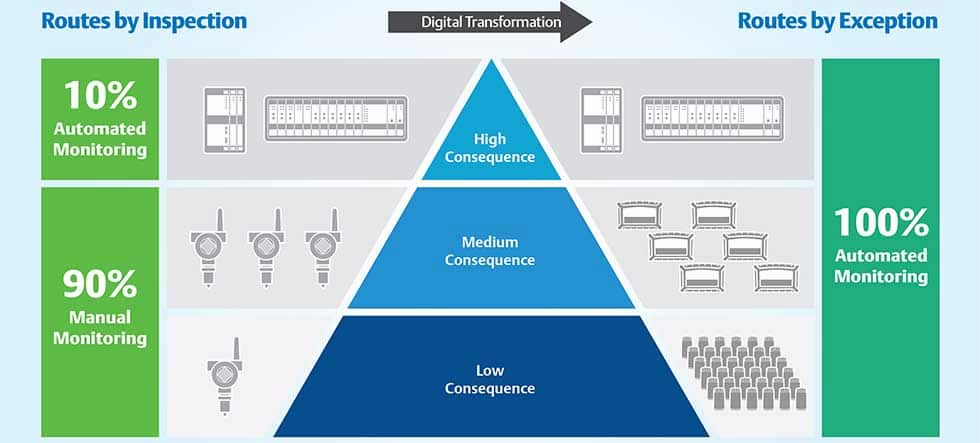
Analytics at the Edge
If a plant’s personnel are freed from walking around the plant collecting data but must spend countless hours analyzing it, the plant still is not operating at full efficiency. To combat this loss of efficiency, many plants are looking beyond wireless sensing devices only capable of delivering raw data. Forward-thinking organizations are instead choosing automated sensing devices with embedded analytics capability alongside basic raw data collection. Advanced edge analytics devices give the plant the option to quickly see and decipher asset health but also provide the necessary raw data analysts need to dive into data for trending, reporting and root-cause analysis.
Online monitoring from rack-mounted systems remains the primary monitor for the most critical assets in the plant. However, routes by exception organizations are monitoring medium-consequence assets using multichannel sensors with built-in analytics users can install and have up and running in hours.
Edge analytics devices collect both vibration and process data from medium-consequence assets to identify the most common faults associated with many assets, such as cavitation or lack of lubrication that can lead to seal damage in pumps.
Advanced monitors can connect to assets using a variety of sensors including pressure, temperature, vibration, tachometers, voltage inputs and more. Using wireless or wired transmission, these monitors alert users to problems and deliver advice via a web interface in real time.
Low-consequence assets can also be brought into the fold with compact wireless vibration monitors capable of delivering full vibration data, accurate diagnostics and prescriptive analytics advice over a self-organizing wireless mesh network. Devices like wireless vibration monitors are cost-effective solutions in this category that can be installed in minutes by plant personnel.
For the most efficient and comprehensive monitoring of plant equipment, many plants are engineering edge analytics devices to report back to a single collaboration portal able to send alerts and advice directly to users’ mobile devices. These tools can apply process data analytics to detect abnormal events and performance deviations to enable decisions for the production process.
Predicting Instead of Reacting
With key health data automatically analyzed and delivered to the palm of a user’s hand by field equipment, plants can detect problems with a balance of plant assets before they impact production, making it easier to move to a “routes by exception” model. Instead of regularly going to the field to collect data, personnel only head to an asset when they know there is a problem—before it would have been discovered using manual data collection. The person traveling to the asset already knows what is wrong and what is needed to fix it. Teams can work more effectively, making informed decisions about the assets they support, while the plant improves visibility, efficiency and availability.