Why condition monitoring might be the right maintenance strategy.
Voith Digital Solutions
07/19/2018
Ensuring driveline availability, efficiency and performance is of the utmost importance in the oil and gas industry. Unplanned downtime caused by equipment failure and decreased performance can seriously impact profitability as well as human well-being. That is why monitoring strategies are crucial in reducing costs while increasing machinery reliability, ensuring product quality and facilitating safety. However, there are many different monitoring strategies to consider, and it is important to understand the advantages and disadvantages of each when selecting the right option for specific machinery.
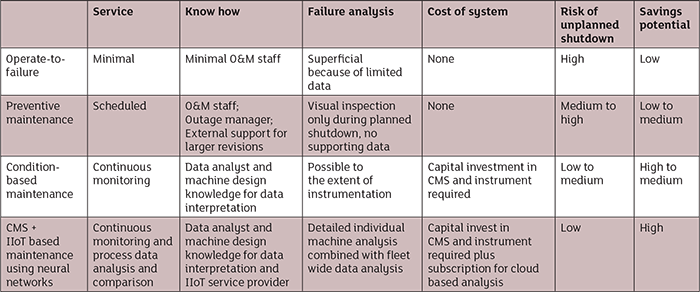
Example
Variable speed gears are commonly used in the oil and gas industry to control mechanically input pump speeds, compressors and similar machinery. The design of the gears allows for quick return on the investment and reduces costs due to energy savings. Typically, the life cycle cost breakeven point is reached within a few years. That said, protection of a total breakdown of the high value asset can only be realized if the speed gear is regularly diagnosed on failures. This may be on an intermittent schedule or continually using a permanent condition monitoring system. In both strategies, it is important to acquire salient signals to identify and emphasize growing failures over time. Analyzing global characteristic values according to standards and guidelines may not provide enough information to identify a failure (example: if a failure is due to the physical characteristics of the structural network of the machinery). Only the usage of advanced diagnosis techniques for failure identification can effectively protect complex machinery. Speed gears typically capture absolute housing and relative shaft vibration permanently. The control calculates global characteristic values to trip the machine over a certain vibration level. Although minor input forces or weak transfer characteristics from forcing source to the receiving transducer may not lead to a high vibration level, the developing damage could be severe. These developing characteristics can potentially be identified using advanced diagnosis tools, provided that the service cycle is shorter than the failure developing cycle. A maintenance strategy with the right time interval can help protect high value assets and help plant owners optimally realize safety and machine reliability.Maintenance Strategies
One of the simplest maintenance options is operate-to-failure, which is running the machine until it breaks and only fixing the problem when it fails. The one advantage to this is more usage of the maximum operating time between shutdowns. However, disadvantages are clearly overwhelming, particularly in work safety with the risk of catastrophic failures. In addition, damage to further drive train components can cause serious consequences in production loss and repair costs. The next level of maintenance strategy is preventative, where regular maintenance is carried out in intervals to ensure the lowest likelihood of failure between repair shutdowns. This strategy results in much greater use of spare parts, as well as more maintenance work than necessary and therefore, increased costs and downtime. In order to decrease downtime and reduce costs, plant operators have demanded an alternative strategy where shutdowns are only scheduled when warranted. This is known as condition-based monitoring (also known as predictive maintenance). This has obvious advantages compared to operate-to-failure and preventive maintenance, but does require having technology available to determine current conditions and to provide reasonable predictions of remaining useful life. Condition-based maintenance (CBM) is usually based on intermittent monitoring, where machine health is inspected by qualified personnel, or continually using permanent condition monitoring systems. With the Industrial Internet of Things (IIoT), the concept of CBM can be improved to enhance machine availability. This can be accomplished by using advanced analysis tools based on cloud computing technologies. Cloud computing monitors the machine condition 24/7 and relieves pressure on engineers and diagnostic personnel by acquiring pre-alarm information automatically and remotely from anywhere in the world. Typically, plant operators use a mix of maintenance strategies that can change significantly over the equipment life cycle. The optimal mix of maintenance strategies is key in adding value to the total cost of ownership. Considering this, the current maintenance strategy should be regularly reviewed and adapted to the changing conditions and targets.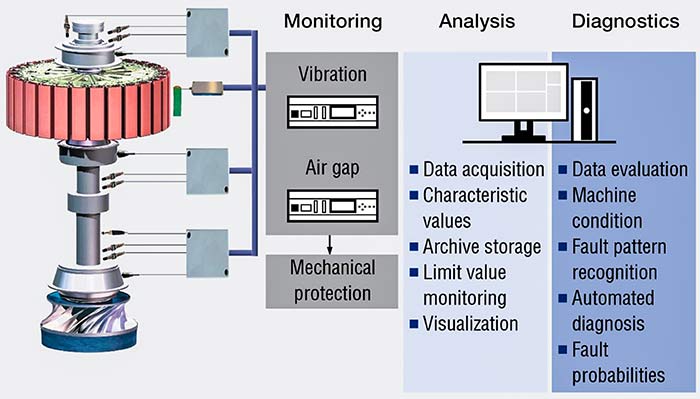
Requirements for CBM & Services
CBM cannot generate money. It only supports savings by two main economic advantages. The first advantage is that CBM does avoid losses of output due to machine breakdown. In addition, it minimizes shutdown time for repair because of the knowledge about the failing machine component. With this in mind, the main question is how much is the capital value of the asset and how much money will be lost due to the outage of the machine during failure? Performing CBM is a balancing act between the expected savings and profit of the plant. Once it is determined that machinery should be monitored on a periodic basis (permanent or intermittent), the next question is: How often is monitoring needed? The period for observing machine condition must be smaller than the amount of time it takes for faults to develop in machinery. Although, figuring out the degradation rate may not be as simple, especially on complex machines depending on many different parameters. Traditionally, the knowledge of the degradation rate on typical wear parts is experienced by plant operator’s maintenance personnel and may even deviate from manufacturer specifications due to installation and ambient conditions.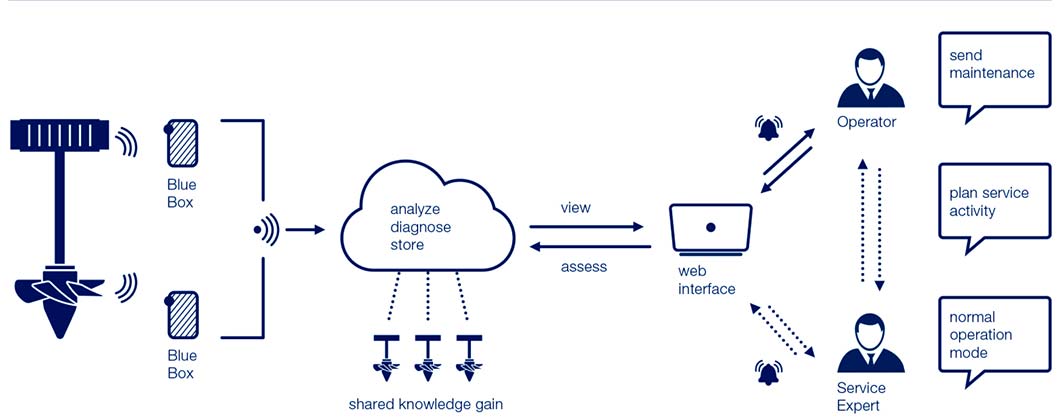
Additional Considerations
Regarding high value assets where unplanned shutdown time is costly or where breakage may negatively affect employees or the environment, permanent monitoring systems may be considered. Below are further advantages of 24/7 monitoring:- statistical analyses on measurement signals to optimize process to ensure or sustain high product quality
- provision to realize optimum spare part management of assets
How to Understand, Analyze & Interpret the Data
Keep in mind that monitoring 24/7 does result in a large volume of data and requires personnel trained in analyzing and interpreting the data. The key challenge resulting from this investigation is when to put gained knowledge to use. Interpretation of signals and the timing of when to schedule repair is difficult and requires experience. Traditional software is able to make only simple decisions like fault localization based on sensor position and peak to peak value evaluation. Today with new software tools, we are able to do far more than that, including differentiation of faults based on known fault patterns, root cause analysis, residual lifetime prediction of components, driveline optimization and automatic reporting. Therefore, the usage of new software tools can significantly support the decision making process for machine owners. Skills in instrument expertise, machine knowledge and digital signal processing are necessary in gaining physical understanding of the process. To comprehend the physics, it may be a requirement to study mathematical models to gather the system behavior and distinguish between correct and faulty operation processes of machinery. To increase autonomous support in terms of interpretation and timing of repairs, artificial intelligence (AI) may support data analysts and process experts in root cause analysis and decisions. However, AI based on neural networks only can interpolate and not extrapolate on training sets (fault condition), meaning that a fault condition must be known to the AI. At this point, big data may come into play. Big data gathers machine faults on similar input variables on whole machine fleets generating those training sets. Over time, this establishes a robust assistant to monitor and diagnose machine failures.- protects and optimizes equipment
- extends operational life
- avoids unplanned downtime
- increases maintenance strategy effectiveness while reducing costs
- increases employee safety.