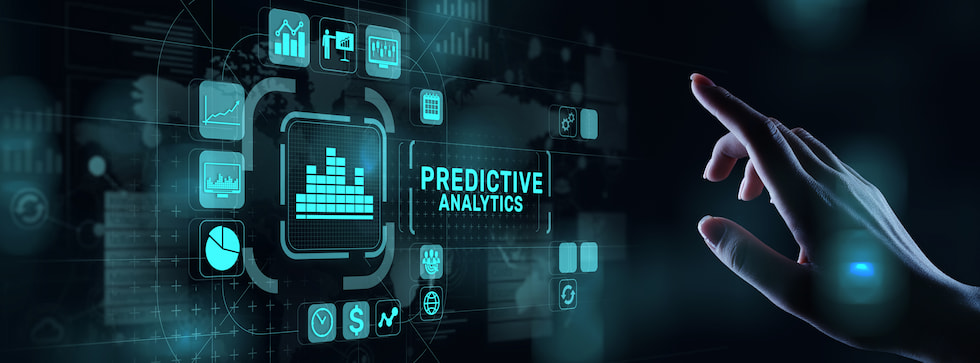
Users do not have to look far to see the promise that predictive analytics holds for the flow control industry. The ability to predict when and why a critical asset will fail—and address the issue before it impacts operations—is compelling. Additional benefits include reducing maintenance expenses and spare parts inventories, increasing energy efficiency and improving plant performance.
What’s not to love?
But predictive analytics is only one part of a complete analytical approach. Sure, predictive analytics might be the shiny, new, exciting buzzword, but without laying the groundwork with a comprehensive analytics strategy, users will never realize all of the offered benefits.
There are three components of a comprehensive analytics strategy. Here is a closer look at each and the roles they play in a holistic approach.
Step One: Descriptive Analytics
What it is: Descriptive analytics includes data aggregation and trending to provide insights into assets’ past performance.
What question does it answer? “What has happened?”
What users need to know: Before a user can predict what is going to happen, a user needs to know what is happening now. Many plants collect this information via scheduled route inspections. This approach offers a snapshot of an asset’s performance at a specific moment in time. The operation gains little long-term insight into how that asset is performing over time.
Installing wired sensors to monitor equipment performance continuously might not be economically viable, given the cost of labor and the disruption to the operation for installation.
One solution that lies in the middle of these is long-range (LoRa) wireless sensors to monitor noncritical assets. Battery-powered, wireless LoRa sensors transmit vibration, pressure and temperature data every 15 minutes, giving the user a detailed description of the equipment’s behavior. Having this historical data can enable users to see how equipment performance is trending. When a performance anomaly is found, users can be deployed into the field to identify and address the root cause.
Manually inspecting only those assets experiencing problems—instead of all assets on a monthly or quarterly basis—can be operationally effective and safer, as it reduces user exposure to potentially dangerous conditions.
This approach also gives users the historical performance data that is needed to move on to the next step: predictive analytics.
Step Two: Predictive Analytics
What it is: Predictive analytics uses statistical models and engineering algorithms to understand an asset’s
future performance.
What question does it answer? “What could happen?”
What users need to know: Now that asset performance data has been collected, users need to review it carefully to learn how certain behaviors could lead to problems. This is where predictive analytics come into play. Machine-learning algorithms must analyze equipment data that contains some peculiar events to be effective; otherwise, they will not learn anything about the flow management system. This period is called the training phase because it trains the algorithms on what to look for based on previous failures.
Engineered-based algorithms developed by flow management equipment OEMs can apply algorithm models based on decades of experience designing and servicing equipment.
For example, pumps often are affected by system-generated problems such as cavitation, excessive pipe loads or thermal transients. These conditions occur less frequently than normal bearing wear, but could cause a significant pump failure. Engineered algorithms use physical laws to identify a failure mode at an early phase.
It is important to emphasize that the quality of the data collected during the descriptive analytics phase has a direct impact on what users can accomplish in the predictive analytics phase. Incomplete or erroneous data will lead to inaccurate predictions.
Step Three: Prescriptive Analytics
What it is: Prescriptive analytics uses optimization, simulation algorithms and experience to advise on the
possible outcomes.
What question does it answer? “What should we do?”
What users need to know: With a comprehensive data collection and analysis approach in place, facilities can turn their attention to understanding what actions they can take to address equipment problems before they lead to downtime or affect performance and solve them once and for all. Prescriptive analytics enables users to determine the best course of action for specific equipment problems.
For example, if predictive analytics algorithms determine a drop in pump flow efficiency, prescriptive analytics suggests the most effective action, such as changing the impeller type or operating the pump in a different condition, to overcome the issue.
A typical prescriptive analytics scenario looks like this:
- Wireless or wired sensors send equipment performance data to an OEM-monitored data center.
- Analytics at the internet of things (IoT) cloud platform evaluate this data to identify anomalies. If the software detects an anomaly, the system alerts an OEM reliability engineer to conduct in-depth analysis.
- The reliability engineer reviews the data, captures additional full-spectrum data from the equipment if necessary, and recommends a course of action based on the data, the expertise and equipment knowledge.
- The user incorporates the changes to address the issues before they impact plant productivity.
A Three-Pronged Approach
Facilities that want to realize the valuable benefits of predictive analytics should take steps to implement a comprehensive analytics program that includes descriptive, predictive and prescriptive measures. This three-pronged approach will generate the data, analyses and recommendations needed to reduce costs, lower equipment downtime and optimize plant performance.