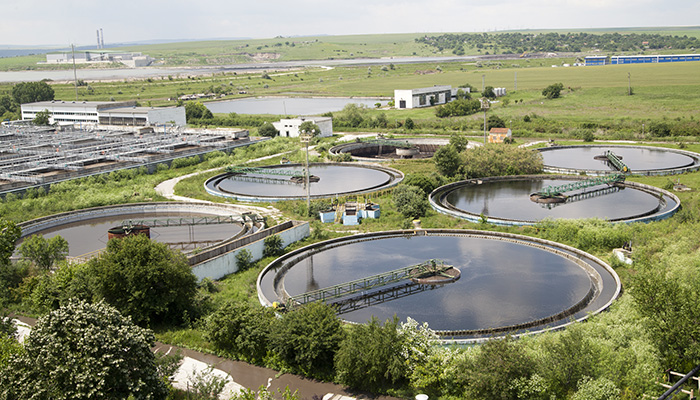
In recent years, meaningful advancements in equipment connectivity, internet of things (IoT) devices and cloud computing have introduced changes to the industry. By leveraging data analytics, these technologies aim to improve operational efficiencies and drive better decision-making. Smart devices integrated to products and equipment enable data collection and subsequent processing and storage via cloud servers.
Within water treatment operations, IoT-based analytics allow utilities and plants to collect data from pumps, water meters, pressure sensors and devices attached to other equipment. That data—which is most often transmitted to the cloud for analysis—is instrumental in generating key insights for enhancing plant operations, anticipating potential issues, conducting predictive maintenance and improving asset management, among other benefits.
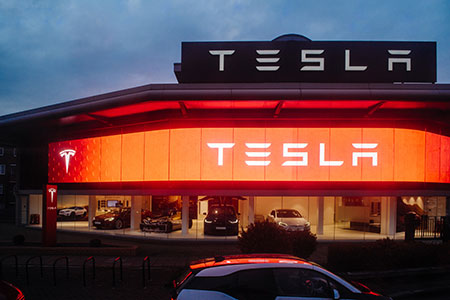
But going beyond the water industry, an increasing number of IoT-based applications—such as autonomous vehicles—require real-time data processing. They cannot afford the lag that occurs with transmitting data between IoT tools and cloud servers. As an alternative to cloud computing, analytics and decisions are now moving closer to the action, where the data is being generated. This is known as edge computing or edge analytics. In edge computing, data is instantaneously processed locally at the sensor level, so decisions can be made much more quickly.
Edge computing is described by global market intelligence firm IDC as a mesh network of microdata centers that process or store critical data locally and push all received data to a central data center or cloud storage repository, in a footprint of less than 100 square feet.
In a report outlining the emerging edge computing landscape, CB Insights—a market intelligence firm that focuses on technology—explains that edge computing reduces the need to transfer data generated by motors, pumps, generators or other sensors back and forth between the cloud, but places more responsibility on the hardware’s underlying edge computing technology.
In addition to faster data processing, the advantages of moving to edge computing summarized by CB Insights include reduced data management costs, lower network traffic, increased application efficiency, and—by de-emphasizing the cloud’s role—reduced risk of a single point of failure. Where cloud computing depends on an internet connection, edge analytics allows devices to operate with real-time control offline, enabling data-based decisions in remote locations and geographies where internet is limited.
Beyond these advantages, the shift to edge analytics can provide specific benefits to water and wastewater operations.
Creating a Shared Data Ecosystem
By minimizing intercommunications with cloud servers and emphasizing localized connectivity, IoT edge analytics introduce opportunities to share data between sensors and devices throughout a plant, creating a more tightly linked water or wastewater operation at a local level.
However, the true benefit of a shared data ecosystem can only be realized if IoT edge tools are allowed to operate based on an open source architecture, permitting different vendor devices in a plant’s supply chain to communicate—sharing downstream and upstream learning for the greater good of the operation.
Device Learning
The artificial intelligence and machine learning behind Tesla’s autonomous driving program is based on what the auto manufacturer calls fleet learning—when one car learns something, they all learn it. Essentially, Tesla’s self-driving cars should be able to continuously improve since its fleet operates as a network and can learn over time from data supplied by the half million cars that the company already has on the road.
In water and wastewater operations, edge analytics enables a similar type of network effect, where the learning acquired from any single device can be pushed across many devices. This can be especially powerful between various devices that are integrated on the same equipment and similar assets.
For example, the learning that can be gleaned from how a reverse osmosis system handles specific water quality conditions, or the way a membrane bioreactor unit responds to certain process changes, can be valuable to operators and owners in terms of informing crucial adjustments and upgrades across all similar installations. Device learning also offers the potential to scale insights and learning to the plant level and throughout an entire organization or geographically across regions.
Security
IoT edge analytics address the security concerns of industries and water treatment plants related to cloud interactions where critical data is sent outside of their networks. Sensitive operations such as pharmaceutical manufacturing, oil and gas, and power generation are uncomfortable with transmitting encrypted data to outside sources due to cybersecurity concerns and the potential of becoming vulnerable to hacking.
As an on-premise solution, edge analytics ensure that data is kept at a local level, with data analytics and data-driven services occurring within the boundaries of the operation or plant.
Creating a Tailored Services Model
For water and process treatment providers, edge analytics promises to improve service capabilities by leveraging localized data to design highly customized treatment solutions. Fine-tuning treatment based on edge analytical learning will also go a long way to helping optimize productivity, minimize downtime, and enhance the reliability and safety of equipment.
Rapidly Advancing Edge Capabilities
Leveraging the power of edge analytics gives companies the ability to capture high quantities of data locally and generate conclusions at the edge without the cloud. However, edge analytics still offers a powerful integration with cloud servers for industries that are comfortable with cloud interactions. For example, companies can publish data points collected from edge devices to the cloud at higher frequencies when certain processes become interesting and of value, providing a much clearer picture. The cloud allows presentation of structured data and smarter trending. Data sets that are consistent from site to site enable better models, which lead to accurate conclusions, allowing changes and optimizations to be made with greater confidence and success.
Conclusions identified from edge analytics can be accepted and trusted on an individual asset basis without the need for a data scientist or an analyst to confirm, allowing automatic changes and optimizations. Likewise, automatic optimizations can be applied to other “like” assets, determined by data set comparisons. As the edge analytics market matures, the role of the data scientist or analyst will shift from examining data and applying changes based on conclusions to designing and monitoring predictive analytics and ensuring that auto-optimizations are effective.