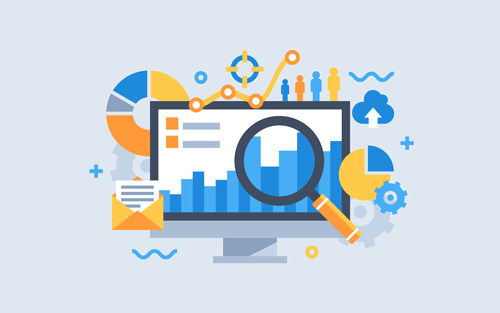
The trend toward predictive maintenance in industrial operations marks a significant shift from traditional, schedule-based approaches to a more dynamic, data-driven strategy. This evolution reflects an increasing recognition of the benefits of utilizing real-time data to anticipate maintenance needs, thereby optimizing equipment performance and longevity. In this context, predictive maintenance emerges as a crucial tactic, pivoting on the detailed understanding of each pump’s unique operational characteristics. Instead of following a generalized maintenance calendar, this method leverages the continuous stream of operational data from the pump. By analyzing this baseline data, predictive maintenance allows for the early detection of potential issues, facilitating timely interventions that prevent larger problems and maintain optimal pump performance.
The foundation of a successful predictive maintenance program lies in a thorough understanding of a system’s initial baseline performance. This critical data serves as the cornerstone for establishing what constitutes optimal operation, enabling users to recognize deviations that signal a need for equipment intervention. By accurately gauging this baseline, maintenance teams can preemptively identify and address issues before they escalate, ensuring uninterrupted operation and prolonging the life span of the equipment. This approach shifts the maintenance paradigm from reactive to proactive, focusing on prevention rather than cure and significantly enhancing the efficiency and reliability of a system.
Understanding Baseline Data in Pumping Systems
The initial startup of new equipment presents a crucial opportunity to establish a baseline for optimal performance. The focus on empirical data is particularly significant in this context. Empirical data is information collected from direct observation or measurement. Unlike theoretical equipment performance data, empirical data provides a tailored, real-time insight into each individual system. This data reflects the unique operational characteristics and environmental conditions of the system, making it an indispensable tool for precise assessment and decision-making.
A critical question is what variables one should select to maximize visibility of the health of pumping equipment. Defining these variables requires a two-pronged approach. First, it is essential to have a deep understanding of the key functional attributes of the equipment, recognizing that these attributes can vary significantly by type and application.
Second, the focus should shift to determining which measurable variables are most conducive to monitoring the life-cycle performance of equipment. These should not only reflect the immediate operational status but also provide insights into long-term wear and efficiency. By carefully selecting these variables, users can gain a comprehensive view of their equipment’s health, enabling proactive maintenance and ensuring optimal performance over the equipment’s life span.
A prime example widely used in numerous operations is the centrifugal pump. Key functional components, such as the impeller, volute and wear rings, significantly influence flow dynamics. As wear occurs in any of these elements, there is a notable shift in the pump’s performance characteristics, including head-to-flow ratios, net positive suction head required (NPSHr), volumetric efficiency and energy efficiency. Monitoring these shifts is crucial for maintaining optimal pump operation.
To track these key performance indicators, one should consistently measure the discharge pressure of the pump, ensuring this measurement is taken upstream of any components that might alter the pressure, such as valves, orifices, filters, etc. Additionally, measuring suction pressure is vital for accurately calculating and monitoring the total head. Combining these pressure readings with flow measurements allows for a real-time assessment of performance degradation over time.
As internal clearances within the pump wear, several changes become evident. The power requirement tends to increase, indicating greater effort needed to maintain the same output. In systems with variable frequency drives (VFDs), an increase in rotations per minute (rpm) relative to flow may be observed, compensating for the reduced efficiency of the pump. Similarly, in systems with control valves, a key indicator is an increase in the valve percentage open for a given flow rate when attempting to maintain the desired setpoint. These changes manifest as a downward and flattening shift in the pump performance curve, signifying a reduced capability of the pump to elevate fluids or work against higher pressures. Understanding and monitoring these variables is key to predicting the optimal time to pull the pump for rebuild or replacement.
Generating an Initial Baseline
Once the critical variables have been clearly defined and the system is appropriately set up for data measurement and logging, the next crucial step is to establish a baseline evaluation scheme. In cases where automation may not be fully implemented, this could involve manual data measurement and recording. However, the ideal scenario is to create a robust process control strategy that incorporates a baseline testing function within the central controller. This function will continuously measure and log a baseline profile, serving as an ongoing reference point for maintenance efforts.
An understanding of max and min values of controllable process variables is critical. When it comes to pumps, their primary objective is typically to deliver a specific volume of liquid against a designated system pressure. This characteristic simplifies the process of defining baseline conditions since manufacturers provide performance guidelines and pump curves that can serve as a foundational framework for establishing a baseline.
A key advantage of creating an initial baseline is that it presents a significant opportunity to capture pump performance tailored precisely to the fluid properties and process conditions the pump was designed for. This means that, during the baseline evaluation, users have the chance to collect data that reflects the real-world operating conditions and unique characteristics of the fluids involved. While performance curves are indispensable tools, the initial baseline serves as a bridge between these curves and the specific fluid properties and process conditions the pump will encounter in its actual application.
Secondary variables, including temperature, conductivity, pH, consistency, viscosity, density and turbidity, as well as electrical parameters like amperage and voltage, oxidation-reduction potential (ORP) and vibrational measurements, are essential for comprehensive baseline monitoring. These variables provide in-depth insights into the system’s performance over time. By consistently tracking these factors, subtle yet critical changes in the system’s behavior can be detected early. This enables proactive maintenance and adjustments, ensuring sustained optimal performance and helping to identify potential issues before they escalate into major problems. These variables, when monitored alongside primary performance indicators, create a detailed and holistic view of the system’s health and efficiency.
In the process of generating an initial baseline dataset, the initial step involves a thorough and detailed measurement of all identified critical and secondary variables under standard operating conditions. This process should be conducted over a sufficiently long period to account for any variations or anomalies, ensuring a comprehensive and representative data set. The goal is to capture the pump’s performance in its typical working environment, reflecting its interaction with the specific fluid characteristics and the surrounding conditions.
For the most effective baseline establishment, utilizing automated data logging systems is recommended. These systems can continuously monitor and record the operational data, providing a rich and accurate dataset. This data then becomes the reference point against which future performance can be compared. In scenarios where automation is not feasible, meticulous manual recording can be employed, although this might not capture the breadth of data as effectively.
Ultimately, the initial baseline dataset forms the foundation for a dynamic and responsive maintenance strategy. It offers a realistic snapshot of the pump’s optimal performance, against which any future deviations can be measured. This proactive approach allows for timely interventions, ensuring the longevity and efficiency of the pump and maintaining the integrity of the entire system.
Using Baseline Data to Build a Bulletproof Predictive Maintenance Program
Utilizing baseline data to shape a predictive maintenance program is pivotal for achieving long-term operational efficiency and reliability. Once a comprehensive baseline data profile is established, the focus shifts to implementing a predictive maintenance strategy that aligns with the specific demands of the equipment. This approach, rooted in continuous data analysis, allows for the anticipation of maintenance needs before issues escalate into system failures.
The key to a successful predictive maintenance program lies in the dynamic use of baseline data. This data, enriched with detailed insights into the pump’s performance under various conditions, becomes a powerful tool for predicting future maintenance requirements. The predictive maintenance program should be designed to constantly analyze this data, looking for patterns or anomalies that could indicate impending wear or failure.