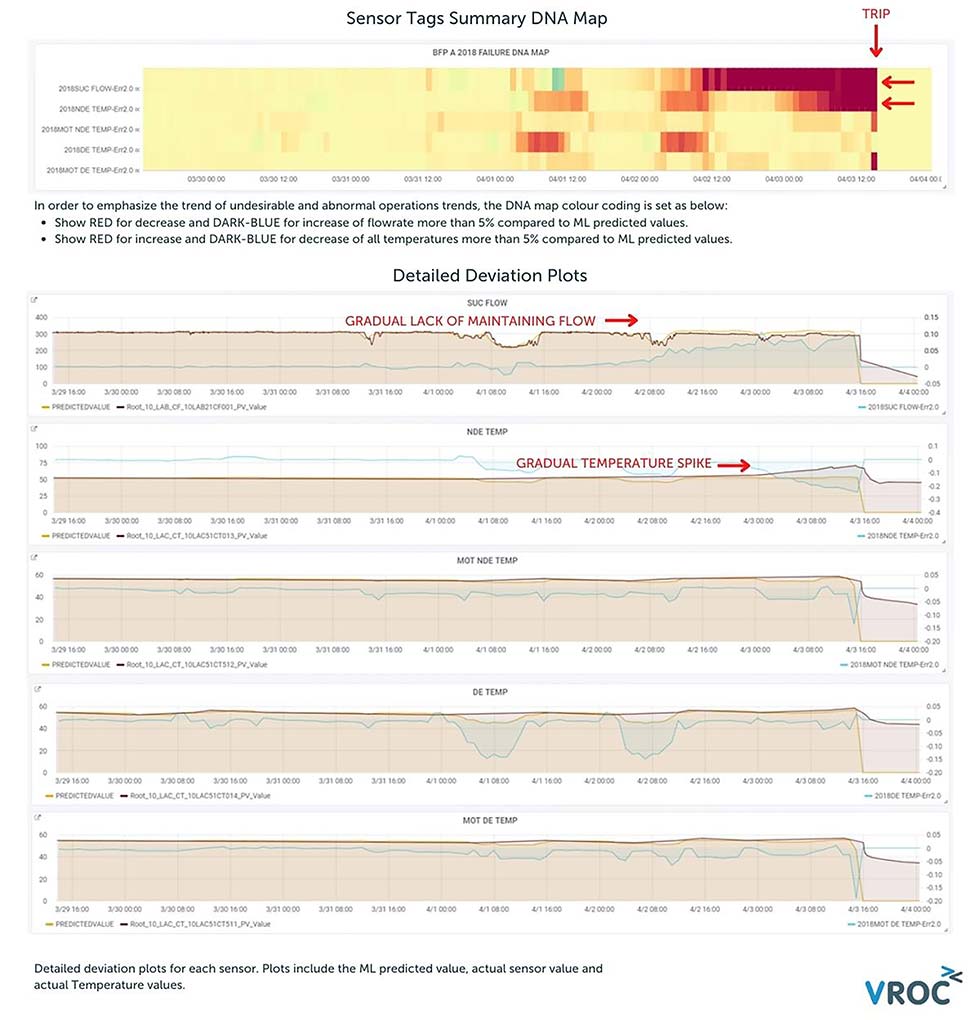
Today, predictive maintenance is commonly accepted as a valid maintenance method, providing data insights for improved asset reliability. Machine learning (ML), a subset of artificial intelligence (AI) technology is showing benefits for predictive maintenance and is now being adopted by industrial operators. This article explores the meaning of machine learning predictive maintenance and how two different operators deployed the technology, assessing its capability for asset health monitoring and predictive maintenance for their pumps.
What Is Machine Learning Based Predictive Maintenance?
Machine learning based predictive maintenance uses equipment sensor data, advanced analytics and machine learning to detect anomalies and predict failures using a risk and/or criticality approach so that maintenance teams can make informed maintenance planning decisions or intervene early to mitigate faults. This method continuously learns and studies patterns leading to anomalies and faults to proactively alert teams as an equipment’s operating envelope deviates from the predicted norm, with the goal of reducing unplanned downtime and improving asset availability and performance.
Machine learning predictive maintenance is different to rules based predictive maintenance, which uses equipment sensor data, condition monitoring and predefined threshold limits to predict when maintenance is required. The challenge with rules based predictive maintenance is that only around 20% of anomalies conform to an expected failure pattern, limiting its effectiveness. Pre-defined threshold limits can result in a high rate of alerts, some of which are false positives that use up valuable resources. Typically, for accurate alerts, thresholds are often only exceeded just prior to a failure, limiting the ability for early intervention.
ML Predictive Maintenance for Coal Power Generator
The following case study was conducted by an AI company and its client—a 200 megawatt coal fired power station in Australia. The purpose of the study was to assess their machine learning capabilities for asset health monitoring and predictive maintenance across their critical assets, including their boiler pumps. The boiler feed pump is a critical asset, as it feeds water into the boiler.
ML models were built to monitor pump health, which included live and historic data for all the critical sensor tags corresponding to pump and motor bearing temperatures, such as driven end (DE) bearing temperature, nondriven end (NDE) bearing temperature, motor DE bearing temperature, motor NDE bearing temperature and pump flow rate. Models are trained on a time period of healthy operation (with no trips or failures) and put into production with live input data allowing the models to predict the asset’s key parameters and observe whether it is possible to identify asset performance and health degradation from normal operation.
The models detected and alerted the team to a gradual NDE temperature increase beginning on April 3 (increase of red in the DNA map meaning actual temperatures increasing against the AI-predicted temperatures). However, the pump suction flow rate model also demonstrated a significant trend of deviation status change from yellow on April 2 to red on April 3, meaning the pump flow rate began to reduce against the model prediction until the NDE bearing failed. It was possible that a misfunctioning bearing could be the result of a change of shaft displacement status and vice versa. This could have potentially caused both the change in the pump curve, which resulted in the flow rate decrease, as well as an increase of friction and possible shaft contact with both DE and NDE bearing housing, which consequently caused the increase in temperature on the NDE bearing, and ultimately, failure.
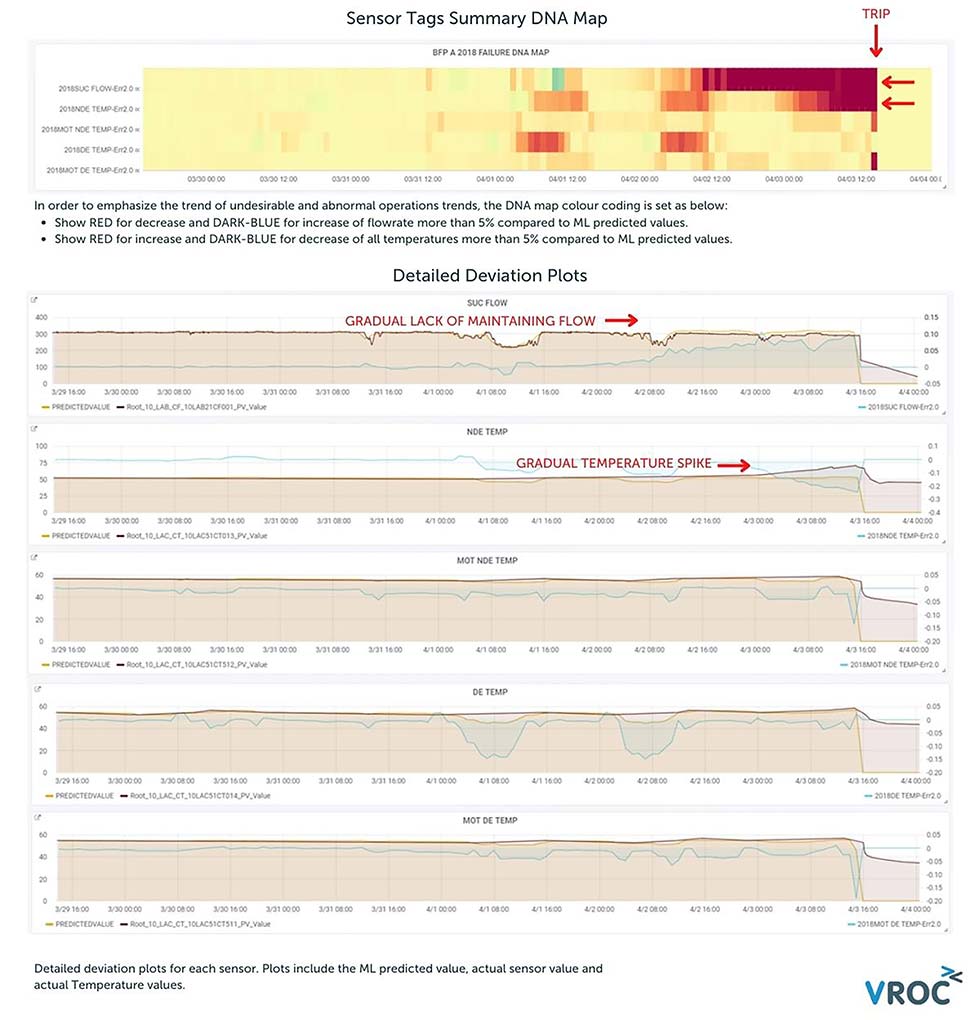
Notes for Image 1:
The models are visualized to clearly show trends of undesirable and abnormal operation trends using both DNA maps and detailed deviation plots. The color code in the DNA map shows red for decrease and dark blue for increase of flow rate more than 5% compared to ML predicted values. They also show red for increase and dark blue for decrease of all temperatures more than 5% compared to ML predicted values. Using the insight acquired from the pump health models, the client was able to react in a timely manner to ensure the availability of a subsequent pump to mitigate downtime. The estimated savings due to the prediction of the failure and the deployment of a back-up pump has been estimated at $150,000.
Produced Water Pump ML Predictive Maintenance for Oil & Gas FPSO
The following case study was conducted by an AI company and its client—an oil and gas floating production storage and offloading (FPSO) located in the North Sea. The purpose of the study was to assess their machine learning capabilities to address specific reliability issues on rotating equipment which were impacting production.
ML models were built using two years of historical data from over 30,000 sensor tags, and the models were put into production with live streaming data to produce predictive asset health dashboards for major rotating equipment, including the produced water pumps. The models for the produced water pump showed significant deviation from normal operating conditions, in terms of the NDE bearing temperature and pressure (Image 2) informing the operation team of a leaking NDE mechanical seal.
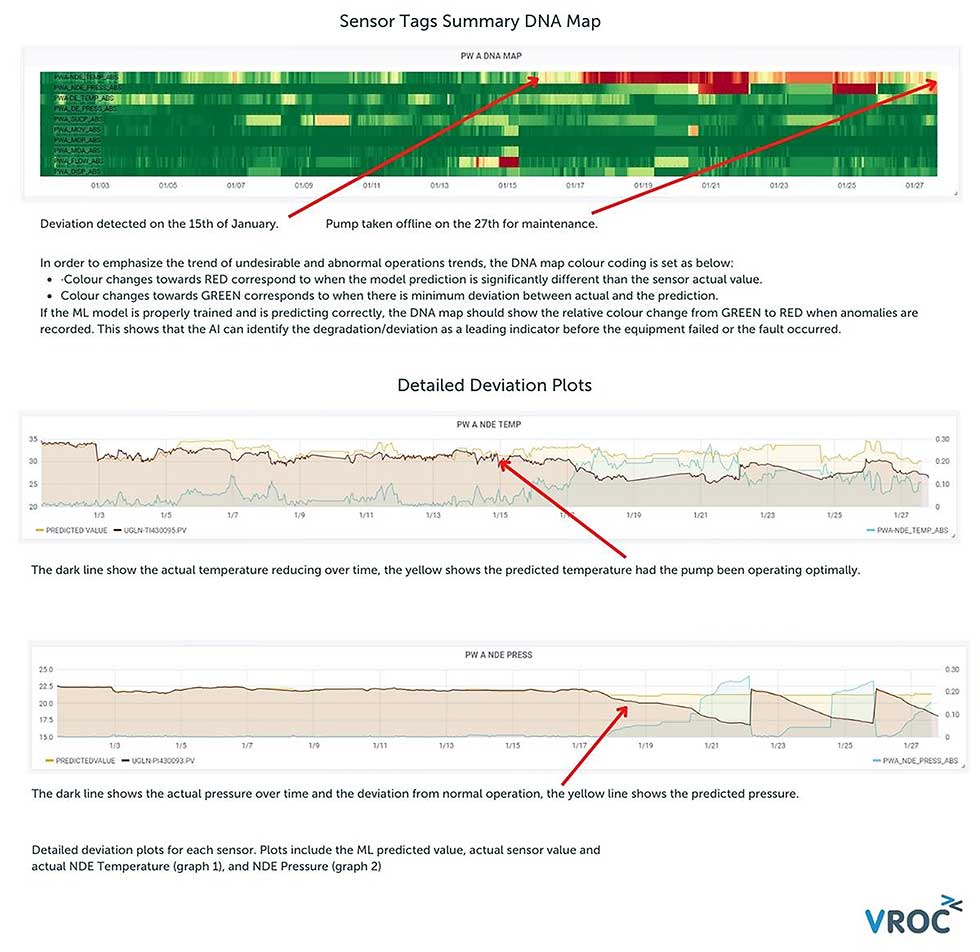
The ML models successfully identified the first failure to occur on this pump—a mechanical seal failure—five days in advance. The reliability team were able to schedule a pump changeover before the mechanical seal failed, ensuring the produced water system could continue operation, preventing a complete plant shutdown. Upon investigation, they confirmed that the DE bearing of the pump had a problem with lube oil leakage. The dashboard showed the temperature dropping, accompanied by the pressure drop, which meant the lube oil was leaking and entering the external surface
of the bearing slightly causing the temperature to drop.
The client confirmed a savings of 30,000 British pound sterling (GBP) per incident to repair if caught early. With potential for a produced water plant trip, saving 500,000 to 1,000,000 GBP (production rate at time) if not caught in time, the end user was able to proactively plan maintenance on the pump.
These examples show the capability of machine learning models to provide accurate predictions of asset degradation and asset failure. Machine learning models provide predictions not limited by predefined thresholds or bias. The advance warnings benefited both the power generation and oil and gas reliability teams, who had the advantage of time to plan necessary interventions to ensure production continued.