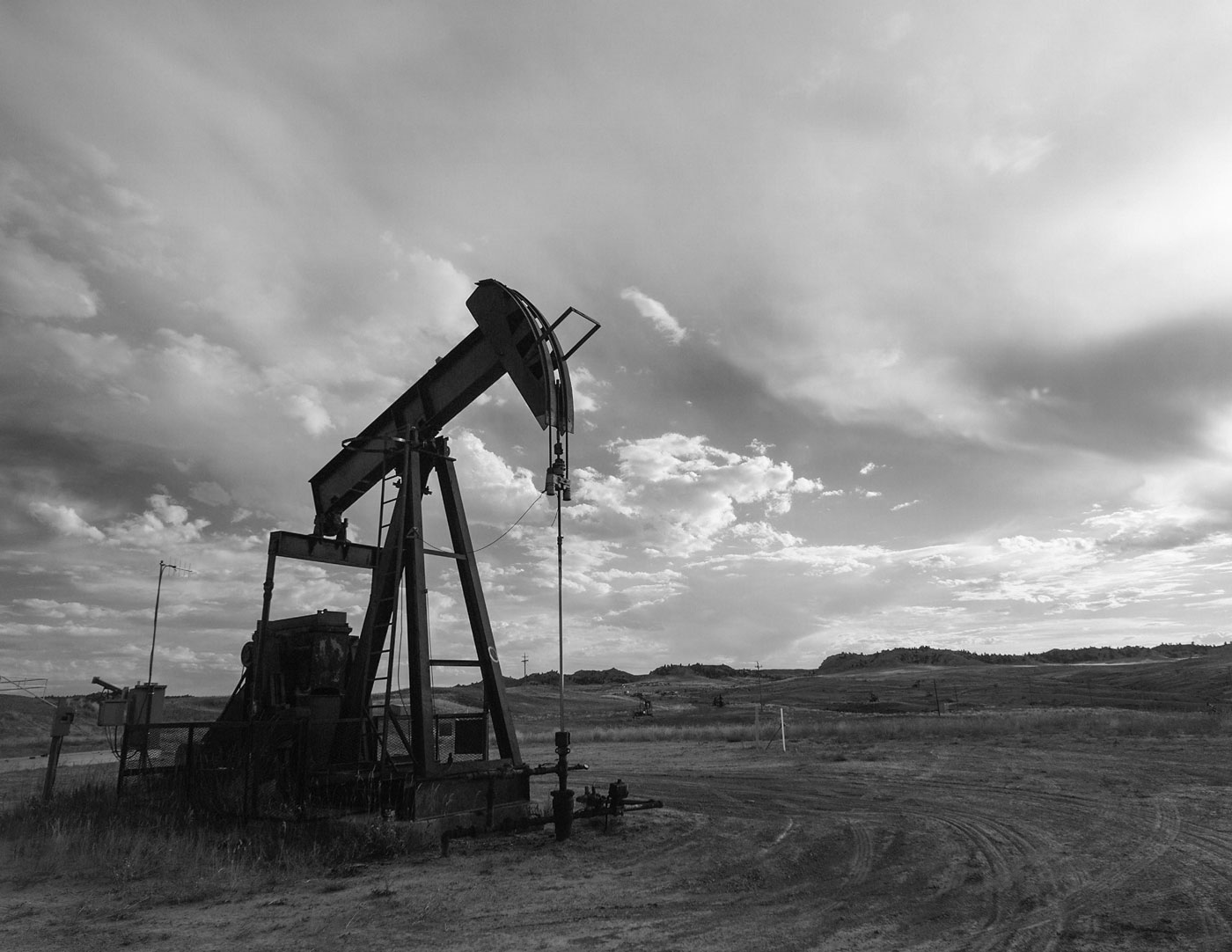
Pumps are vital to industries including water treatment and wastewater facilities, power generation, oil and gas, food processing and more. In the oil and gas industry, the uptime of industrial pumps is especially critical. The total world consumption of global petroleum and other liquid fuels averaged 92.30 million barrels per day in 2020, according to the U.S. Energy Information Administration. That total has risen by approximately 5 million in 2021 and will continue to grow in 2022. Any unplanned downtime can impact the ability to meet this growth.
There are three basic types of pumps, and they are classified by how they transport fluid: positive-displacement, centrifugal and axial-flow. Pumps can experience several different types of failures, including cavitation, bearing failures and seal failures, among others. In oil and gas, conditions in which pumps operate are often challenging, dirty and hazardous, resulting in wear and tear. Failure of these pumps not only results in unexpected operation delays and increased costs, but it can lead to dangerous oil and gas leaks, impacting labor safety and the environment. To avoid these unexpected failures, many companies increase preventative maintenance and create aggressive inspection schedules. These practices, however, can sometimes lead to unnecessary part replacement, maintenance costs and labor.
Others may rely on condition-based maintenance, which focuses on maintenance performed after monitoring real-time data and detecting unacceptable condition levels. However, this may not come with the advanced warning needed to prevent impending failure events or avoid downtime. By taking a predictive approach, past maintenance data and current sensor measurements can be used to determine early signs of failure, allowing companies to perform maintenance only at the exact time it is needed.
.jpg)
Predictive Maintenance Challenges
Developing and deploying a predictive maintenance solution for pumps is challenging. It requires a combination of sensing and instrumentation expertise, domain knowledge, and a practical perspective on applying machine learning and analytics for predictive monitoring. The instrumentation aspect is crucial since this data will be analyzed and will serve as the foundation of the actionable information. The decisions made from this information include what maintenance actions are needed and when they should be taken given the current pump health, as well as any trends or patterns that could emerge.
Vibration is typically the most crucial signal to use for monitoring the condition of a pump, but information on the rotating or reciprocating motion is also useful, especially for performing the more advanced signal processing methods. In addition, pressure and flow rate measurements are important for understanding pump operation and providing context for understanding the vibration data. A balance must be struck between the benefit of including these important measurements versus the hardware and implementation costs of doing so. This challenge is especially true for vibration sensors. Domain expertise is needed to place a minimal set of sensors to keep the hardware cost down and monitor the pump properly and accurately.
When handling the analytics, it is challenging to apply machine learning for this application without any domain-specific preprocessing and signal processing steps. Typically, pump failures are rare, so using a supervised machine learning model is not typically practical. Instead, a combination of domain-specific feature extraction methods for the vibration signals coupled with a baseline-based anomaly index machine learning algorithm is a more reasonable approach. The deployment and user interface should be closely aligned with the industrial use case and expected user, as well as the problem being solved. For some applications, it is not feasible to transmit the data to a remote monitoring center or central server, requiring the analytics and deployment to be performed closer to the data source.
Additionally, the frequency of data collection and the speed of both the data analytics processing and the reporting of asset health information would vary based on the industrial application and use case. These aspects should be evaluated during a preproject phase. By following requirements and best practices, solution developers can formulate the appropriate approach and the solution that should be considered and ultimately implemented.
.jpg)
Case Study
A global oil and gas contractor with a specialty in automated drilling equipment and rig components wanted to develop a health monitoring solution for its mud pumps in the field. The contractor wanted to reduce unplanned downtime and unexpected failures. Not only did the company want to prevent these failure events, but they also wanted to distinguish between anomalies caused by maintenance issues and anomalies due to sensor issues.
By working with a predictive analytics company, this client sought to differentiate these anomalies, address the pump failures, and validate the solution by utilizing the induced fault data collected on its test rig.
A Predictive Maintenance Solution
The user provided the analytics company with a year’s worth of historical data from test bed data sets and sensors on the piston, suction and discharge mechanisms on two pumps in the field. The team of analytics experts was able to pull crucial features from the data by considering vibration patterns in the frequency and time-frequency domain. These features were integral to the development of health assessment models. The models then helped determine key indicators of pump seal failure, as well as establish the accuracy and necessity of the sensors.
By using advanced signal processing and vibration-based pattern recognition, the health monitoring system was able to detect and diagnose pump failures. This solution provided a baseline health assessment, failure identification and pattern recognition diagnosis capabilities.
Outcomes
The predictive analytics company was able to identify potential issues, as well as establish the best locations for sensor placement. The final solution predicted mud pump failure at least one day in advance, providing the data needed to take action and proactively perform maintenance. This approach helped reduce downtime, increase productivity, improve safety and prevent leaks.
Getting Started
- Criticality analysis is essential in order to select the pumps for which predictive maintenance solutions can best be applied and to choose a solution that can provide the most value.
- After determining the target pumps, the most critical failure modes should be identified, along with any relevant maintenance records for unplanned and planned downtime.
- Determine what data has been collected, if any, and review what data could be available from the pump’s controller.
- Based on the data and common failure modes, determine sensor placement and what, if any, additional sensors need to be added to the monitored pumps for the predictive solution.
These initial steps are essential when partnering with a technology provider and can help companies develop and adopt a predictive maintenance solution for their pumps that is robust and accurate.