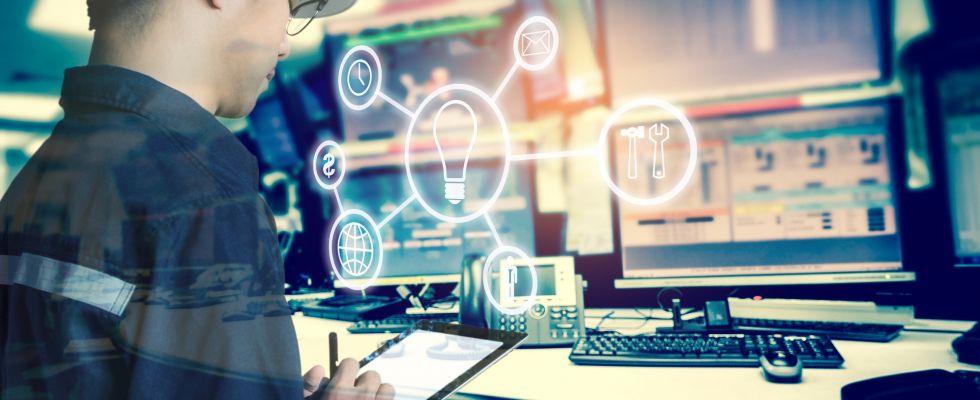
Increasing financial flexibility to safeguard against commoditization can be obtained by getting as much out of existing production capacity as possible, and one way to do that is by applying advanced analytics to individual, yet critical, assets such as pumps. The people managing those types of assets who have begun to understand the importance of embracing new technologies stemming from the rise of Industry 4.0 are realizing the value that digitalization can bring when they are fully able to leverage the volumes of data at their disposal on their own, without the need for a data scientist.
These forward-thinking organizations in the processing and chemicals market are turning to solutions like self-service analytics to create an analytics-enabled workforce, not only to strengthen their market position and create the most profitable factories of tomorrow but also to address critical production issues and make better informed decisions.
Advanced Analytics & Hubs
Due to emerging monitoring and analytics technologies evolving at a rapid pace, there are self-service analytics platforms now available that can empower users with the key information needed to translate their time-series data into value. Within these self-service predictive analytics platforms, there are dashboards, or specialized hubs, that make it easier and less costly to manage the maintenance of assets like pumps and avoid equipment malfunctions or failures.
In fact, pumps are better monitored today than ever before. This shift toward operational excellence is being powered by dashboards that alert users about any potential failure before it happens. Customizable hubs can be configured to specifically manage complexities using advanced analytics to solve potential issues and also optimize performance. Users can maximize what they can do with their data in context (contextual data) to get a deeper insight into what is happening.
For instance, this can be done by using a repository combined with a search engine and collaboration component, so items in context can be aligned to assets such as pumps as well as other processes and events.
In the pump-heavy chemical processing sector, the platform can be configured to organizational requirements for not only pumping but also all chemical processes. The result is that the context becomes a new influential dataset that is both visualized and analyzed side by side with the time-series data. The context can be used as a real-time monitor for potential pumping issues and process failures.
Potential Pump Challenges
Chemical pumps need to be constructed out of materials that are able to withstand higher levels of viscosity, corrosiveness and abrasiveness because that can be one of the many reasons a pump could fail. Model-free advanced analytics delivering time-series data can help with preventive and predictive maintenance.
In other words, process behavior can be more readily monitored, and trends of key data viewed and accessed more quickly and conveniently. Using self-service analytics leads to fast root cause finding and action-taking when pump issues or failures arise.
Chemical metering pumps provide flow measurement and control for applications and benefit from a hub to ensure the precise volumes of chemicals are being used. Such a hub safeguards and provides alerts for any operating problem within the pump fluid transfer system.
In fact, there are many issues worth monitoring that could impact a pump’s performance such as excessive vibration, premature bearing failures, operating temperature increases and cavitation issues. With progressive cavity pumps, dashboards can ensure the correct flow speed and can help prevent problems with reduced suction capabilities, increased wear and premature failure.
Another type of pump worth noting is the centrifugal pump because of the frequency of its use in chemical applications. This is where such a hub can help by monitoring the levels of pressure that is impacting the centrifugal force, potential overheating due to low flow and leakage along the rotating shaft.
Advanced analytics can also help with ensuring certain codes, standards and regulations are met and compliance is maintained. In many cases, pumps need to meet or be certified to such standards that comply with either ASME, ANSI, API, NACE, etc., before they can be used in certain boilers, pressure chambers or other chemical applications.
Beyond pumps and other assets, advanced analytics are certainly used by chemical companies to monitor production, address complex issues and extract information on processes without lengthy data-modeling or analytics experts.
Four Different Types of Analytics
Data analytics is necessary for any organization to measure and track its process data and make informed decisions on their operations. Gartner Research lists four different types of analytics.
These four types generally grow in complexity, but all serve a purpose within the value chain of analytics on assets (pumps and other equipment), processes and events.
1. Descriptive analytics:
What is happening in my operations?
This form of analytics is the most common and perhaps the simplest, but nonetheless vital. It provides the insights and key metrics needed to visualize and understand plant operations. Think of descriptive analytics as the “who, what, when, where, how/how many” of basic reporting. With self-service analytics, engineers can visualize their operations to fully understand what is happening at all levels.
2. Diagnostic analytics:
Why is this happening in my operations?
Diagnostic analytics are used to uncover the why of an event and then determine the root cause of the problem. This is a useful form of analytics when dealing with time-series data because it helps engineers understand the cause and effect between various data sources, as well as identify patterns. With a pattern recognition-based self-service solution that is responsive and intuitive, process data can be analyzed and interpreted. That is the key to closing the analytics skills gap and bringing advancements to where it matters: the process experts.
3. Predictive analytics:
What will happen in my operations?
Predictive analytics take things a step further. This form of analytics uses plant data, and the findings from descriptive and diagnostic analytics, to predict specific outcomes based on trends and dependencies. Predicting anything from equipment failure to production evolution, predictive analytics allows decision-making that could have a giant impact on operations.
Historically, predicting the behavior of batches had been accomplished by building complex data models that were hard to build, costly to maintain, and the results were hard for process experts to interpret. New approaches, such as self-service analytics, are available that are fast and iterative, easy to interpret and robust. Process experts using self-service analytics can tackle predictive analytics independently, leading to, among other things, debottlenecking central data science departments.
4. Prescriptive analytics:
What do I need to do in my operations?
This final form of analytics takes all plant data, insights gathered and trends produced, and determines potential future courses of action. Prescriptions require a good diagnosis, so it often comes down to predescribed suggestions that allow manufacturers to take appropriate actions on the notifications they receive and the instructions provided by the system. With every new situation, knowledge expands for fast and appropriate action when early warnings are sent or needed action is predicted by the system. This is called user-driven machine learning. In a complex chemical environment with small batches, for example, this is crucial.
While these types of analytics are important to optimizing pumps and reducing downtime, robust analytics can also deliver tremendous value across all operations in production—positively impacting the plant engineer, maintenance engineer, safety engineer and/or production engineer.
Empowering most of the plant employees with key data really means making business intelligence and analytic tools accessible to all so that they can simply and easily derive new insights just by using their particular expertise, without needing to acquire sophisticated analytics skills.
This is best described as the democratization of analytics.