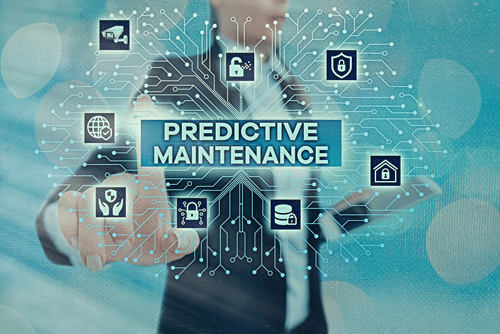
Predictive maintenance has emerged as a significant advancement in maintaining the efficiency and reliability of industrial assets, including pumps. By leveraging advanced data analytics and machine learning techniques, predictive maintenance programs can identify potential issues before they become critical, reducing downtime and maintenance costs. Artificial intelligence (AI)-based predictive maintenance solutions offer a more comprehensive and holistic approach, enabling pump engineers and maintenance teams to make informed decisions and take proactive measures to prevent failures. This article will explore five practical tips for building a successful predictive maintenance program for pumps, delving into the nuances of data collection, model selection, alarm thresholds and other key elements to ensure success.
1. Comprehensive Data Collection: The Foundation of Predictive Maintenance
The first step in building a successful predictive maintenance program is to gather comprehensive data. This involves not only collecting data related to pump performance (e.g., vibration patterns, temperature and flow rate) but also needs to consider external factors to the process that may impact operations. By continuously collecting and analyzing
this data, users can identify trends, patterns and deviations that indicate potential issues.
It is sometimes possible for AI-based solutions to detect and predict issues with equipment despite a lack of relevant sensor data being available. This can be seen in the case study of an oil rig generator failure, whereby a temperature model reacted to a problem from a cooler system, predicting the failure of the generator. Even though the cooler system lacked remote sensing, the AI model could still predict the failure. With sufficient and accurate data, AI models can reliably predict the root cause and time to failure. These critical insights can help maintenance teams intervene in time to avoid downtime and failures.
2. Model Selection: Tailoring AI Models to a Pump System
Choosing the appropriate machine learning algorithms for a predictive maintenance program is crucial for accurate and reliable predictions. Different models excel in different scenarios, so it is important to evaluate the problem statement and data set and select the most suitable model for the pumps. As most pump engineers are not data scientists, no-code, AI-based predictive maintenance solutions offer an ideal workaround. Pump engineers can simply select their preferred model outcome, such as anomaly prediction, forecasting, time-to-failure, degradation or root cause analysis.
In this case study, an engineering team produced AI models to see when their water supply pump was degrading. Using the insights obtained, the team was able to identify the root cause of an issue with
their pump and plan and implement necessary maintenance seven days later without any downtime, resulting in a savings of up to $250,000.
3. Alarm Thresholds: Balancing Sensitivity & Specificity
Rules-based predictive maintenance programs are heavily reliant on threshold alarms. As such, the setting of appropriate alarm thresholds is a delicate balancing act. The threshold should be sensitive enough to detect potential issues early on but specific enough to avoid unnecessary downtime due to false alarms. By defining specific thresholds, users can minimize the number of false alarms and ensure maintenance activities are only initiated when necessary.
An AI-based predictive maintenance approach uses equipment sensor data, advanced analytics and machine learning to detect anomalies using a risk and/or criticality approach, helping maintenance teams make informed decisions. This method learns by studying the patterns that lead to historic anomalies and faults and continues to study new data to uncover future trends.
An example of this can be seen in this case study where an engineering team for an oil platform in the North Sea used AI models to detect the deviation of two sensors related to the bearing vibrations of a gearbox. The model helped the team uncover a previously unknown critical speed bandwidth that was causing excessive vibration and damage. With this information, the team were able to mitigate the failure. Had the team not had an AI model analyzing the data, they would not have been alerted to the issue, and a failure would have been likely.
4. Identifying Root Causes With a Holistic Approach
Traditional maintenance programs often focus on addressing symptoms rather than identifying the root cause of equipment failures. Predictive maintenance programs, on the other hand, aim to detect and address the root causes of issues. By taking a holistic approach to analyzing all available data, including critical equipment sensors, industrial control systems, ERP systems, computerized maintenance management systems and production systems, users can identify the underlying causes of pump failures and take preventive measures.
An oil and gas platform in southeast Asia had a moisture problem unfolding with risk of a gas leak. The team created
an AI model to help identify the root cause of the problem. The model analyzed the platform’s live data and concluded a gas well was the leading indicator of the moisture problem. The gas well was a separate process entirely to the moisture analyzer; however, due to a holistic approach to data analysis, the model was able to correctly identify the issue, and the team avoided an environmental incident, saving close to $1 million. By embracing a holistic approach, engineers can identify hidden patterns and correlations that might go unnoticed with a narrow focus.
5. Integration With Maintenance Workflow: From Prediction to Action
Predictive maintenance is not just about predicting failures; it is about taking timely actions to prevent them. Integrating the predictive maintenance program with the existing maintenance workflow is crucial. Engineering and maintenance teams need to work closely together and share critical information so maintenance schedules and the ordering of spares can be optimized.
An AI-based predictive maintenance approach can be adopted by both brownfield and greenfield sites without historical asset failures. For example, it was used to detect the first ever failure on a produced water pump with a five-day lead time. This information equipped the maintenance team with the right information at the right time to plan their repair and schedule a pump changeover before the asset failed.
Predictive maintenance is all about providing data lead insights to operations and maintenance teams for increased efficiency and cost reduction. Building a predictive maintenance program for pumps requires planning, data analysis and the implementation of appropriate technologies. By following the five tips outlined, users can ensure their predictive maintenance program is efficient and effective. By utilizing AI-based solutions, users can leverage advanced analytics techniques, gain valuable insights and optimize their pump maintenance practices.