Much like a chain on a bicycle or a chain used to pull a lodged vehicle out of a ditch, the value chain of the internet of things (IoT) is only as strong as its weakest link. Often the results or lack of results are due to breakdowns in the people and process link of the value chain. Take, for example, monthly based predictive maintenance routes, where the vibration technician goes to each pump or asset, connects the sensor, collects the data, goes to his or her office, analyzes the data and, if a problem is detected, writes up a report or work order. In many cases, the work order is completed and a larger failure and cost of maintenance or lost production is avoided. It is also common for the work order to be delayed for more urgent work. The nature of a work order from a predictive or leading indicator is that the machine is still running. When the work is delayed, it is typically due to misunderstanding the report. In these cases, the report is vague, uses terms or vibration jargon unfamiliar to the maintenance decision-maker, or simply says, “Do this repair at your next earliest convenience.” All too often there is not a convenient time, the repair is not prioritized, the repair is not completed, the pump fails and the value of predictive monitoring is not realized. The breakdown in this value chain is in the people and process link. The sensor was connected, the data was collected, the analysis was completed, the work order filled out, yet the process of prioritizing and completing the work was broken.
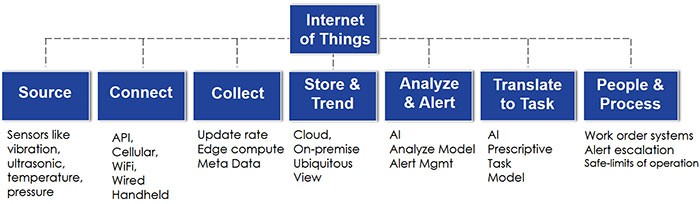
How the 7 Elements of IoT Help
Based on decades of experience in the industrial maintenance space, the first way the seven elements of IoT help is by defining the necessary links to get all the way to “value” or work completion. In past years with or without IoT, the common failure point in creating value from predictive maintenance has been in the people and process link. Many route-based or wired predictive monitoring deployments omitted this element. With luck, value may have been delivered initially when there was a lot of attention to the effort, yet over time the value was lost, perhaps because of personnel turnover, or a lack of a well-defined or understood “value chain.” In starting new, or reviving predictive maintenance programs, one of the first questions to ask is, “When is value realized?” A typical answer is, “When the report is delivered, or when the alert is sent.” But, the value is realized if, and only if, the recommended work gets done. In these 7 elements, the work gets done in element No. 7 by the people and the process. The challenge to ensuring a successful predictive maintenance monitoring solution—whether it is IoT or another tool—is to work successfully, consistently and expediently through the seven elements to get to value creation.The Broken Value Chain
Common pitfalls in the rush to use IoT, artificial intelligence and machine learning often involve overlooking one of these seven elements, resulting in a broken “value chain.” For example, many data science companies are working to bring value and the benefits of machine learning, artificial intelligence and predictive maintenance to industry, yet are unfamiliar with failure modes and the effects of industrial pumps. In many cases, the use of anomaly detection creates nuisance alerts and unproductive tasks for the maintenance tech to check on assets or process where no problem is found. In other cases, the baseline methods used with anomaly detection miss existing failures and the new technology and programs take on an immediate hit, scoring one for the unpredictable machine.Here is how to translate the 7 elements of IoT to industry:
1. Source: To establish a strong link for the source element (pump), users need to know what conditions or failures are most valuable to detect. The most common issue for pumps is seal failure. To detect and mitigate this early requires understanding the root cause. The likely root cause of a seal failure is shaft deflection or vibration; the cause of shaft deflection could be cavitation, not running at the best efficiency point (BEP), bearing fault and/or lubrication fault. Selecting a leading indicator or measurement is key to building a strong link for the source element. A best practice for monitoring a leading indicator for seal health is to use ultrasonic and vibration monitoring. Ultrasonic is a less known measurement and is a strong leading indicator of cavitation, bearing and lubrication faults. Vibration is a tried-and-true measurement that detects late-stage issues like misalignment, looseness, pipe resonance or late-stage bearing failure.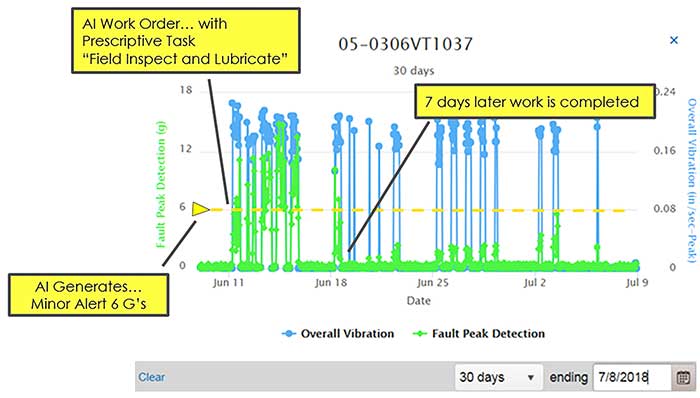