In the global oil and gas sector, failure of pumps, compressors, heat exchangers and other critical equipment systems can have significant consequences for field operations, safety and revenue. Today, most equipment monitoring systems raise static alarms based on high/low thresholds for individual sensor values. These sensors are often inadequate for capturing the wide range of normal behavior of complex oil field equipment systems and unable to separate true potential failures from false positives. Given the substantial impact of pump and other equipment failure, there is a need to complement existing systems using advanced analytics. The convergence of several long-term technology creates new opportunities for failure prediction in critical equipment:
- Data storage and compute capacity are increasingly inexpensive and effectively unlimited.
- Sensors continue to decline in cost and physical footprint.
- Sensor, device and asset-level connectivity continue to improve in quality and cost.
- Machine learning tools and techniques are increasingly accessible and easy to use, even with limited training and resources.
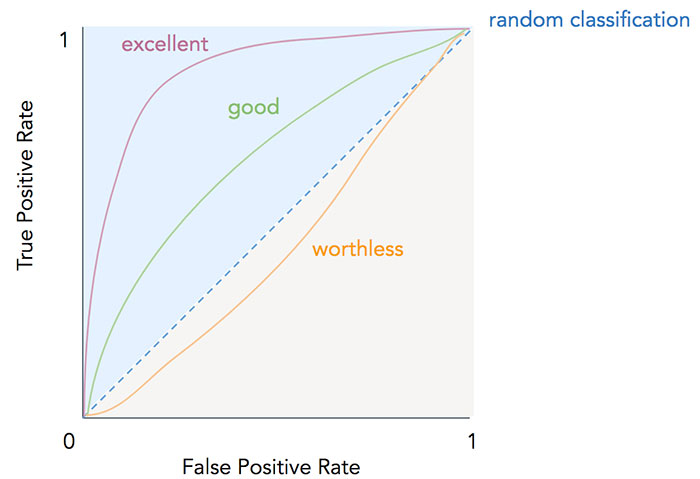
Machine Learning
In oil and gas operations, machine learning models may provide earlier detection of failures and assist engineers and other field personnel in the diagnostic process for anomalous equipment behavior. Critical equipment could contain anywhere from five to 100 sensors. Typical threshold-based alerts look at individual sensors in isolation; these are inflexible and could lead to false positives. The goal of advanced anomaly detection is to create threshold alerts based on a more complex view of equipment behavior that distinguishes among numerous types of normal operating modes. This significantly improves the fidelity of any resulting alerts. For instance, one particular method of anomaly detection can learn behavior based on historical sensor data. With sufficient data, the algorithm can differentiate among typical operating modes (such as ramp-up, ramp-down, or various normal operating behaviors) by creating groups, or clusters, of sensor values over time. Modern software can consider multidimensional clusters that are typical of today’s equipment, accommodating dozens of inputs as frequently as once per second or faster. Machine learning models such as this provide an early warning of abnormal equipment behavior with an indication of where the behavior is outside normal envelopes, allowing an engineer or maintenance personnel to troubleshoot and diagnose failures quickly and efficiently. In one recent instance, an anomaly detection model for critical oil field equipment was tested against status quo maintenance practice to determine predictive value based on known historical failures. The model raised an alert two weeks in advance of status quo maintenance practices for an actual failure event. It also identified the most anomalous sensors as the pressure and flow sensors of the system, indicating the most likely sources of failure. In this particular event, advanced anomaly detection would have resulted in nine days of additional production, reducing the total lost production by 40 to 50 percent, a potential impact of $12 million to $16 million for this field.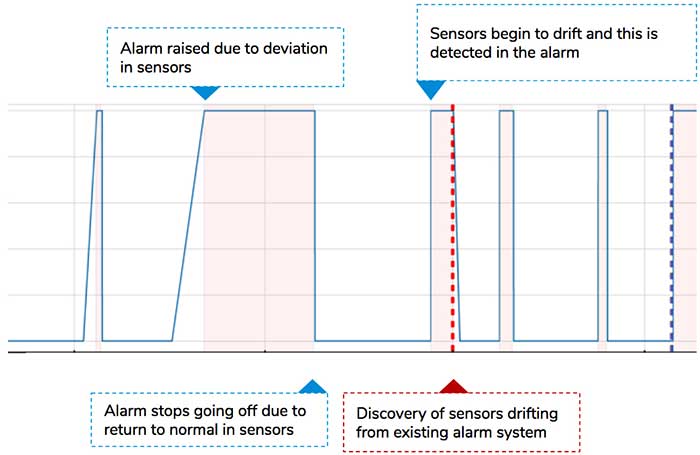