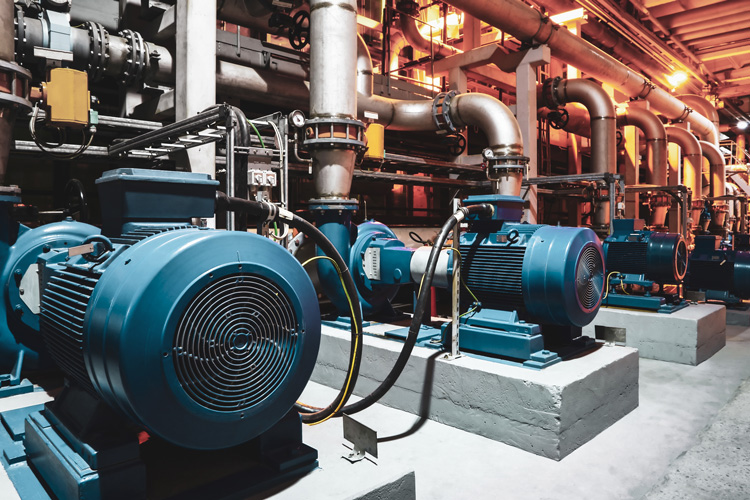
Monitoring the condition of bearings installed inside motors, pumps and other balance-of-plant rotating assets has commonly been accomplished by trained professional vibration analysts—aided by complex software and a portable data collector. Using the route-based technique, the analyst will usually walk a predetermined path within the plant, stopping at each machine and taking readings of vibration data using an accelerometer and the above-mentioned collector. After the route is completed, the collected data is uploaded to a host computer, where, typically, a more experienced analyst reviews and analyzes the data to determine if there are any machine faults. This condition monitoring method produces large amounts of data, requires (usually several) human experts to operate, has time constraints, and is prone to missing important machine faults if not performed consistently. Notably, significant time can be spent on machines with no problems. Of course, additional safety considerations, such as having personnel exposed to the potential hazards of rotating machines, are also in play.
Thanks to advances in technology, particularly the advent of the industrial internet of things (IIoT), condition monitoring can now be automated with the use of wireless, battery-powered vibration sensors that are permanently installed on balance-of-plant assets. These systems use cloud-based platforms for data storage as well as artificial intelligence (AI) or machine learning algorithms for establishing vibration alarm limits and machine fault diagnostics. This automation frees up the vibration experts from routine data analysis and allows them to focus on more problematic, high-profile assets.
Discovering a Machine Fault: Typical Scenario
After spending most of a day taking vibration readings on multiple machines in a plant and returning to the office to review the data, a vibration analyst notices the analysis software is indicating high vibration on a bearing in the driven end of a centrifugal pump. Vibration is high in both radial directions but not in the axial direction. Performing a fast Fourier transform (FFT) spectral analysis on the vibration waveforms indicates some vibration at the machine’s 1X running speed but well below alarm limits. But some high vibration peaks are showing up at nonsynchronous (not harmonic to the 1X running speed) frequencies. The analyst knows from experience this is a possible indication of a bearing fault developing, but additional analysis is needed to confirm this.
Looking up the pump’s bearing part number in the plant’s computerized maintenance management system (CMMS), the analyst then pulls up the bearing’s characteristic fault frequencies—fundamental training train frequency (FTF), ball spin frequency (BSF), ball pass frequency outer (BPFO) and ball pass frequency inner (BPFI)—in the analysis software. The analyst notes the characteristic BPFO lines up close to a vibration peak in the FFT. In addition, there is a harmonic at twice the frequency of the vibration peak (at 2X BPFO). From experience, the analyst knows this is confirmation that the bearing has a defect in its outer race. Further measurements in coming days will confirm the severity of the bearing fault and how soon the bearing should be replaced. The analyst’s recommended corrective action should include ordering a new bearing and scheduling time to shut the pump down to replace the bearing.
Did this scenario meet the goals of a condition monitoring program? Yes. The analyst was able to detect a bearing problem early, take corrective action to fix the problem and, thus, avoid unplanned downtime.
But there are a few things to note in this scenario:
- At least one trained vibration analyst is required to operate this system.
- This entire process was manually executed and labor intensive.
- Vibration data is stored locally across both a portable data collector and the plant’s network infrastructure.
- It is not clear where or how vibration alarm limits were established.
- The machine fault diagnosis was manually determined, relying on the analyst’s skill and experience.
- Confirming the severity of the fault requires focused attention on the machine, including repeated daily measurements that are outside of the scheduled routine measurement route.
- Although not mentioned, how often are measurements taken? Once a month? Could the machine fault have been discovered earlier if readings were taken more often?
Automating Condition Monitoring
A wireless condition monitoring system involves the deployment of battery-powered, wireless vibration sensors on the plant’s rotating assets. These sensors communicate with a gateway that, in turn, is connected via a cellular network to the internet. Data from the sensors is sent to the cloud, where sophisticated analytics process the information. Users will then be notified of machine faults via a web-based dashboard on a PC desktop or push notifications from a phone app. With a wireless, IIoT-based system, many aspects of a conventional condition monitoring system can be automated, enabling data to be collected all day, every day—without fail.
What Expertise Is Needed?
In a nutshell: Users do not have to be trained vibration analysts to operate a wireless condition monitoring system. Basic knowledge of machinery and where best to install the vibration sensors on the machines is needed. Once the sensors are installed, the system runs in the background, monitoring the plant’s machines.
This frees up the vibration analyst’s time to focus on problem machines. In a route-based program, the analyst spends a large amount of time taking readings on machines that are fine. This is because all machines must be checked regularly, as no assumptions can be made about the machine’s condition. With a wireless system, the condition of a monitored machine is known at any given time. This allows the analyst to zero in on machines flagged with a fault.
Where Is the Data & How Is It Protected?
In a conventional condition monitoring system, vibration data is first collected in a portable device and, at the end of a collection route, uploaded to a host computer. This means the system user must provide the requisite computer infrastructure, which can require installing a complex server and involving corporate information technology (IT) personnel. In any event, at any given time, data is spread from a portable device to a corporate network. Because the data is often moved around manually, risk of data loss—ranging from physical damage to the portable unit to corporate network failure due to cybersecurity threats—becomes a serious concern.
With an IIoT-based approach to condition monitoring, a wireless system does not need a local server or local host. Nor is data scattered across multiple devices. Vibration data is measured in the sensor and transmitted to a gateway, where it is periodically uploaded to a cloud-based platform via a cellular network. Data is stored, processed and managed in the cloud. The end user only needs a so-called “thin client” device—such as a web browser, tablet or phone app—to view the data.
What About Alarm Limits & Fault Diagnosis?
Appropriate vibration alarm limits not only protect machines, they allow the analysts to prioritize the problematic machines. Accordingly, it is important to set realistic alarm thresholds.
But how do alarm thresholds get set? Users could start with the International Organization for Standardization (ISO) 10816 recommended levels, but this standard’s machine classifications are often not appropriate for the machine in question and can produce unrealistic alarm levels (too high or too low). What about a machine manufacturer’s recommended levels? Often these do not consider actual operational realities, which applies especially to older equipment. Consequently, alarm thresholds tend to be set by experienced analysts, although this can take much trial and error to accurately dial in.
In contrast, an IIoT-based wireless system employs AI that incorporates machine learning algorithms to automate the setting of alarm thresholds. This means the system learns the machine’s behavior during a training phase—while the machine is in actual operation. And if the machine is repaired or overhauled, thus changing the operational behavior, the user can simply reinitiate the training phase.
In a conventional system, a trained professional vibration analyst can diagnose machine faults—assuming sufficient time and appropriate data are provided to perform the analysis. In a wireless system, however, machine fault diagnosis is automatic because the vibration data is being processed in the cloud with every measurement. Rolling element bearing fault diagnosis provides perhaps the best example of this use of AI, particularly with respect to detecting failures in their early stage. Corresponding to each bearing part number are the characteristic fault frequencies that are provided by the bearing manufacturer. When the wireless system analyzes the vibration waveform’s spectrum, it will look for vibration peaks that match those characteristic frequencies. And while a human analyst can do this too, an IIoT-based wireless system performs this analysis automatically.
Data Granularity
As discussed, a conventional route-based condition monitoring system consists mainly of an analyst taking measurements on machines along a preplanned route. But how often does the analyst restart the route and return to the same machine? How often is a vibration reading taken on the same location on the same machine? More frequent readings will yield smoother data granularity, whereas less frequent readings will produce a coarse granularity. While many analysts aspire to repeat the route and take readings on the same machine each month, experience tells us this does not always happen. Perhaps the analyst gets pulled away on a special project or, for whatever reason, decides to skip that route this month. As mentioned, if a machine is flagged as problematic, the extra time and attention this machine needs may take the analyst out of their regular route schedule. Anything that causes measurements to be missed creates blind spots in the data. Meanwhile, what is happening to the machine between measurements? Can the analyst determine a machine fault now, knowing that data on the machine may not be taken again for several months?
A system with permanently mounted wireless vibration sensors takes data readings multiple times per day, every day, which eliminates blind spots in the data. This results in an efficiency gain, as many machines run fine and do not require the analyst’s attention. When the system does flag a faulty machine, the analyst can
focus on that piece of equipment, knowing the rest of the machines in the plant are being monitored.
Advances in technology, including the development of cloud-based IIoT devices, have given wireless vibration-based condition monitoring solutions an advantage over conventional, route-based systems. Because a professional vibration analyst is not needed to operate the system, their time is freed up to focus on problem machines.
Moreover, an IIoT wireless system is cloud-based, which minimizes the risk of data loss and removes the need for the end user to provide a computer infrastructure to store and process vibration data. Furthermore, the analytics built into the cloud platform enable automated vibration alarm thresholds and machine fault diagnosis.
But perhaps the biggest advantage to a wireless system is the permanently installed sensors that take data readings every single day. This is in contrast to a route-based system, which can leave blind spots in the data and makes the analyst guess the condition of the machine in between measurements.