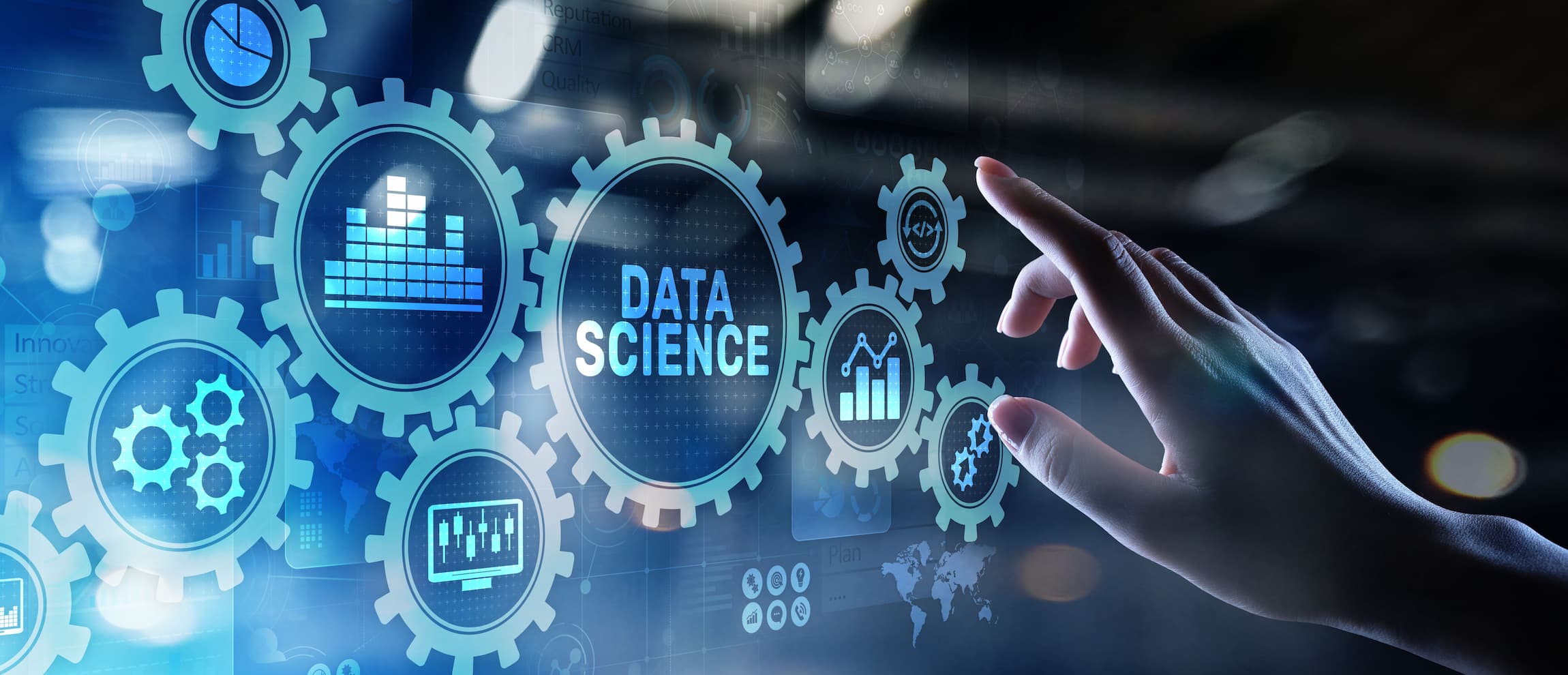
The industrial internet of things (IIoT) produces massive amounts of data at record speed, some reports saying 100 times the volume and 1,000 times the velocity that enterprise resource planning (ERP) systems generate data today. The sheer volume, variety and velocity of this data can be overwhelming, especially to industrial companies, many of which may struggle to translate big data into meaningful and effective operational improvements. If good people are looking at good data, that is a waste of time. They should be looking at the problems and solving them.
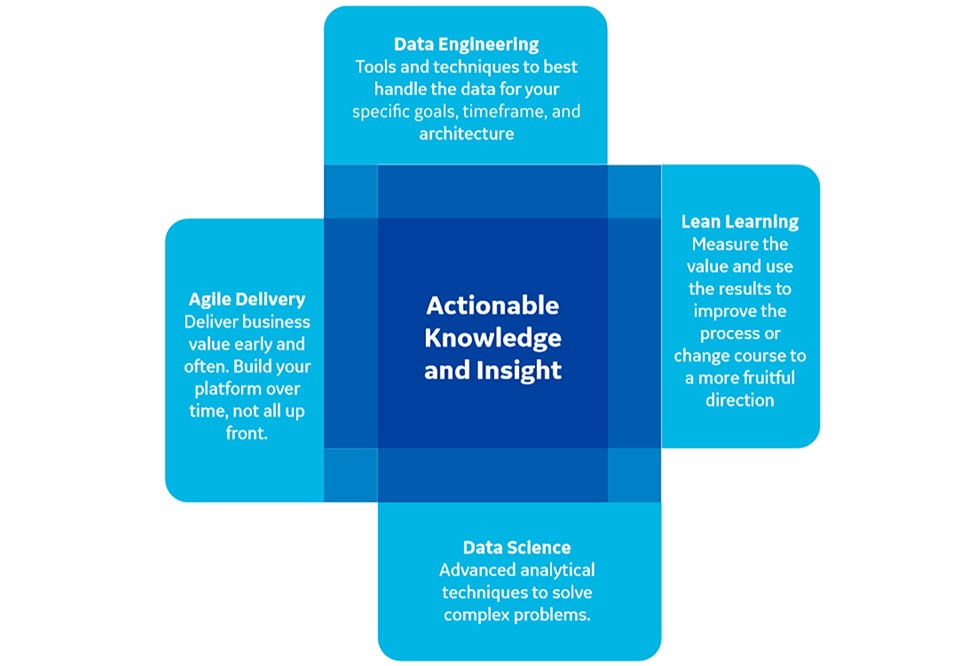
That is where data science comes in. Data science is at its best when it is built in the context of core operational work processes and functions, so the value of any analytics derived from data science can be integrated into daily operations and companies can better operate, analyze and optimize their business processes.
Data science is the art of analyzing data and applying scientific principles and mathematical tools to uncover key patterns within the data that drive significant business value. Data scientists can help build custom analytics that addresses a specific problem, or set of problems, within an organization. Leveraging data science and its techniques such as machine learning, an algorithm’s ability to gain insight from data patterns, can further drive value by providing clearer insight into massive, confusing and siloed industrial datasets.
Data science as a practice has surged in the past five years due to advancements in tools and technologies. In addition, companies are realizing the complexity of dealing with large amounts of disjointed data systems in their organizations and are challenged to figure out how to generate more value from the data they have or continue to capture.
The benefits of data science include:
- Turning data into insights and actionable, tangible business outcomes.
- Increasing throughput, improving quality or solving other common problems within manufacturing sites with data-driven root cause analysis.
- Building solutions for predictive analytics and data-driven management.
- Improving field service operations through scheduling optimization.
- Predicting failures before they happen by understanding data patterns.
- Identifying corrective measures with prescriptive models.
- Transforming empirical knowledge into mathematical algorithms that can be operationalized through IIoT solutions.
More companies are embracing IIoT to drive better business outcomes, but they still are not making the most of the data they collect. The following examples showcase how data science can help solve some of the biggest and most common problems industrial organizations face with big data.
Traditional Monitoring & Visualization
Challenge: Users need to make sure their systems operate properly and take appropriate action (raise alarms, create work orders, etc.) as soon as there is abnormal behavior or failure in the system or its components.
Solution: Many companies monitor system components and report failures as part of their standard operating practice. One way around the manual monitoring approach is to implement business rules, which are logics defined by domain experts to detect abnormal behavior in a system and take proper actions when needed. However, a challenge with this approach is that people do not have the analytic capacity to simultaneously take hundreds and thousands of parameters into account while also considering every edge case. Also, these rules might be contradictory and could therefore end up generating numerous false alarms and target misses.
This is where data science and, more specifically, machine learning can help. Instead of trying to define business rules, the data science team can use a machine learning algorithm that will process the data coming from the system and separate failure operating conditions from normal ones. Algorithms may produce differing levels of detection accuracy dependent on the specific domain and corresponding data, and data scientists will explore and compare the accuracy to suggest the best approach for specific needs. Some data science experts will also incorporate physical modeling into anomaly detection to further improve the quality of outcomes needed for industrial applications.
By using machine learning practices to automate the monitoring of processes, subject matter experts can review the outcomes and confirm or reject the failures that were detected. The machine learning algorithm will also learn from operator input, improving accuracy.
Prediction & Forecasting
Challenge: Downtime costs are a major factor in business. Is there a way to predict how the system will work under future performance parameters and forecast any potential failures?
Solution: A predictive approach should be considered instead of relying on the reactive strategies used in traditional monitoring and visualization methods. By feeding data into a predictive model, users can simulate the relationship between multiple variables and, therefore, forecast how the system will perform under a variety of conditions. This approach also allows the staff to take proactive action to manage future requirements for incoming events.
Predictive diagnostics can determine an asset’s health in real time—accurately predicting its performance to suggest corrective action before problems occur. This method allows companies to connect disparate data sources and use advanced analytics to turn data into insights while fostering collaboration and knowledge management across the organization. The result is less downtime and more control over asset maintenance and performance.
System Reliability & Survival Analysis
Challenge: Real-world equipment reliability can be different from the parameters provided by equipment manufacturers. Is there a way to predict reliability using actual data?
Solution: By analyzing actual field data, machine learning and artificial intelligence can predict the likelihood of equipment failures and warn users ahead of time, regardless of what the manufacturer’s predicted failure rate may be. This allows the team to replace equipment and key system components before they fail, reducing downtime and revenue loss.
Applying Principles of Data Science
In any use of data science, typical outcomes include a collection of insights, including analytics that is useful to the business or stakeholders within the business. Here is how to get started in data science. First, choose the problems that the company will most benefit from resolving.
Second, before investing fully in data science, assess the quality of the data available to develop an understanding of the relationships between data sources. The goal of this step is to ensure that the quality of data is sufficient enough for analysis and that the data has entitlement with the problem to be solved. If there is no value in the data about desired outcomes or key performance indicators (KPIs), the data gaps will have to be addressed.
Once it is determined, and proven, that data is good enough to use in modeling, continue with testing different machine learning algorithms or other modeling techniques to develop a solution. This should allow “citizen data scientists”—users with some domain knowledge and a surface-level understanding of machine learning algorithms—to apply techniques and algorithms and evaluate the results. This will help determine whether the system is truly working more efficiently and if the value justifies the investment in data science.
As is frequently the case in large industrial companies, different departments have access to different data resources with little cross visibility. This needs to be addressed early on because data convergence is a critical component in using data to drive better business decisions. Investigate a solution that allows the analytical framework to easily access representative data samples from a wide variety of data sources along with random sampling, data aggregation, data cleansing, missing value protocols, data normalization, rescaling and more.
Lastly, it is critical to remember that unless data science and its outputs (analytics) are built in the context of daily work, the value of that data science gets lost. Companies should work to ensure their analytics are baked into and made sustainable by whatever technologies they choose to employ. Otherwise, each data science exercise becomes a one-off, siloed project.
With data science that is integrated into daily operations, industrial organizations can derive more value from data and use that intelligence and insight to optimize the performance of assets to increase reliability and availability, minimize costs and reduce operational risk, driving better business outcomes and intelligent business decisions.